Agentic AI uses sophisticated reasoning and iterative planning to autonomously solve complex, multi-step problems.
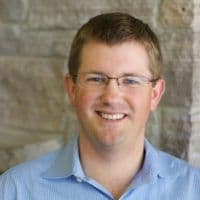
Erik Pounds
January 3, 2025
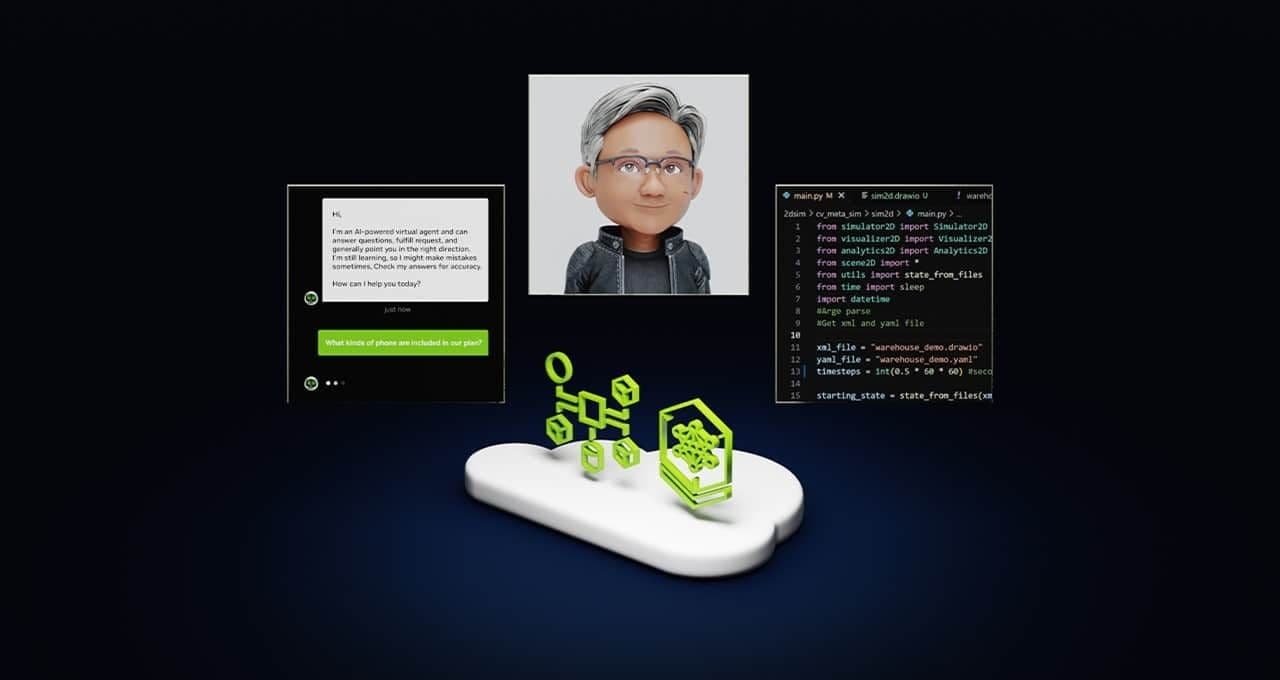
Perceive: AI agents gather and process data from various sources, such as sensors, databases and digital interfaces. This involves extracting meaningful features, recognizing objects or identifying relevant entities in the environment.
Reason: A large language model acts as the orchestrator, or reasoning engine, that understands tasks, generates solutions and coordinates specialized models for specific functions like content creation, vision processing or recommendation systems. This step uses techniques like retrieval-augmented generation (RAG) to access proprietary data sources and deliver accurate, relevant outputs.
Act: By integrating with external tools and software via application programming interfaces, agentic AI can quickly execute tasks based on the plans it has formulated. Guardrails can be built into AI agents to help ensure they execute tasks correctly. For example, a customer service AI agent may be able to process claims up to a certain amount, while claims above the amount would have to be approved by a human.
Learn: Agentic AI continuously improves through a feedback loop, or
“data flywheel,” where the data generated from its interactions is fed into the system to enhance models. This ability to adapt and become more effective over time offers businesses a powerful tool for driving better decision-making and operational efficiency.
Across industries and job functions, generative AI is transforming organizations by turning vast amounts of data into actionable knowledge, helping employees work more efficiently.
AI agents build on this potential by accessing diverse data through accelerated AI query engines, which process, store and retrieve information to enhance generative AI models. A key technique for achieving this is RAG, which allows AI to tap into a broader range of data sources.
Over time, AI agents learn and improve by creating a data flywheel, where data generated through interactions is fed back into the system, refining models and increasing their effectiveness.
The end-to-end NVIDIA AI platform, including NVIDIA NeMo microservices, provides the ability to manage and access data efficiently, which is crucial for building responsive agentic AI applications.
Agentic AI in Action
The potential applications of agentic AI are vast, limited only by creativity and expertise. From simple tasks like generating and distributing content to more complex use cases such as orchestrating enterprise software, AI agents are transforming industries.